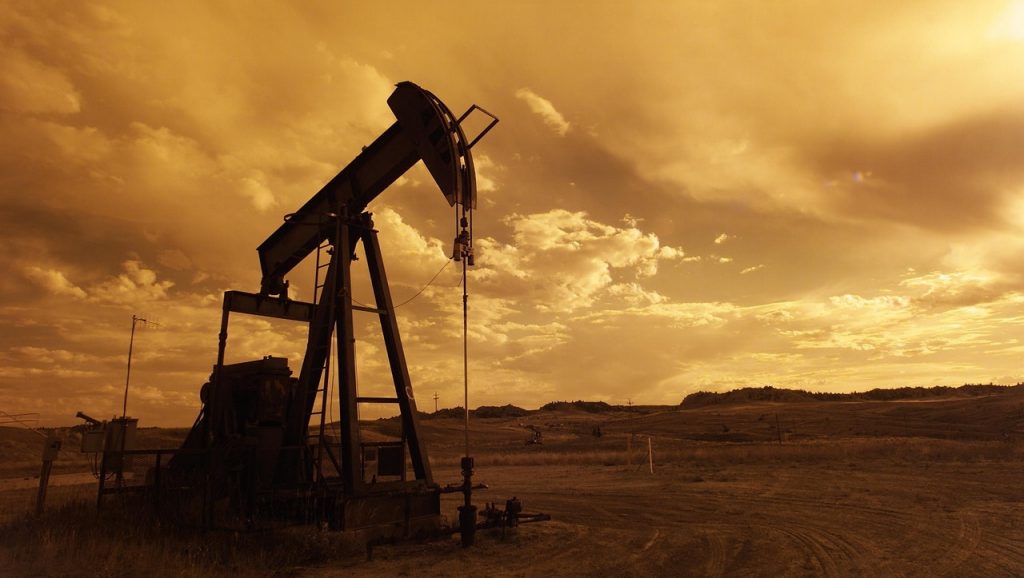
Data as the new oil, but what does that mean? What is the actual value of data? And what does this have to do with corporate social responsibility?
Oil is a resource traded globally. Data is an asset, an asset that grows in value through use, similar to intellectual property. A single industrial site’s data is not very valuable. Combining the data generated by thousands of sites, such as oil pads, is a completely different story. Coupling that with data generated in different operating modes creates new insights and increases the value of data for different stakeholders.
If data is so valuable, then why do you and so many others throw it away?
Some try to determine a price for their data and try to understand what they would get when selling it. But the value in data not only lies in its volume. Uber for example connects customers with a demand. Individual consumers provide data on where they want to get to. Uber uses this to match the consumers to drivers. They also aggregate the data to provide insights into market trends and usage patterns. Uber doesn’t sell data, at least not as their primary service, but they do use it extensively to optimize their processes.
It is not about selling data, it is realizing that data is the lifeblood of an organization.
The value of data to Uber is not captured in a pricing approach. Yes, their data is valuable to third parties, but it is more valuable to themselves as they seek to optimize themselfs. Indeed, without data, they could not continue to operate.
Therefore, we can’t think of data value as simply the market price for selling it. We have to re-imagine methodologies for data valuation. The distinction, from data value to data valuation, is crucial. Data value is an asset. Your data has a certain value and is essential to understand what it is in order to make appropriate investment decisions to create products to extract this data. To understand the value of your data you need a methodology for data valuation. You need a way of working out what the actual value of your data is.
Think of data as an asset; organizations invest in assets to create value for their stakeholders. You have to assess and understand what data you have, then you have to put a value on this data so your teams recognize the value of data, treat it with respect inside your organization and increase its value like a refinery. After this, you have to invest to make sure your data is fit for purpose and ensure you have good governance in place, an appropriate data strategy, standards, systems and procedures to ensure good data quality.
Using the data is about finding out how you can use data to create value for your stakeholders. This may be by optimizing operations. It may be through more efficient delivery. It may be by using the data to generate new insights that are valuable themself such as personalized advertisements. Then you can create value by acting upon these insights. Finally, you have assess what you have learned and how to improve in the future.
You iterate between the data valuation and data value phases. The start is data valuation, something that no one has been able to properly implement and that is partly why so many data initiatives fail.
The discussion about value is not unique to data. ~1800 Karl Marx and others try to determine value. Adam Smith Bailey argues:
It is essential to value, that there should be two objects brought into comparison. It cannot be predicated of one thing considered alone, and without reference to another thing. If the value of an object is its power of purchasing, there must be something to purchase. Value denotes consequently nothing positive or intrinsic, but merely the relation in which two objects stand to each other, as exchangeable commodities.
(Bailey p. 11)
Bailey is clearly differentiating between intrinsic value in which value is something belonging to the commodity in isolation from other commodities, apart from and prior to exchange, and a relative notion of value in which value is only something that exists through the relation of one commodity to another.
Competence Center Corporate Data Quality describes two different approaches as:
Approach | Cost approach: The value of a data object equals the cost incurred for reproducing an exact copy of that object | Income approach: The value of a data object equals the total economic benefit created by that data object in the future |
Key Takeaways | – Considers data quality as a value determinant – Helps manage data quality effectively Helps raise awareness of data as an asset («price tag for data») and quantify a «minimum data value»Is easy to apply and therefore cost-efficient – Allows internal benchmarking Does not take the value created by data into account – Presupposes high maturity of DQ-tools – Provides no incentive for data managers to save data production cost | – Considers data quality as a value determinant – Analyzes one specific process and how data is used therein – Takes the future value created by data into account (e. g. cost savings, risk reduction, increase in revenue) – Is very flexible and scalable – Reveals process inefficiencies – Is highly subjective in terms of being a company-specific and/or process-specific value indicator – Is dependent on process knowledge and/or expert knowledge and therefore not very cost-efficient |
Bottom line in terms of being applicable to data valuation | Delivers valid results, but does not consider the value created by data | Takes the value created by data into account, but is highly subjective and quite costly |
I propose that an organization starts with a clear vision and derives valuable data from there. As a starting point, two main categories should be created. Specific data with strong evidence of high value such as position information of moving parts (especially when the parts interact) and asset usage oriented data such as run time of motors etc. The second category contains all other data, which looks like that there is no information in it but you never know what the future will bring. In a second step assign a number that is adequate not to overemphasize data over stand-alone value. Let’s say 10% of the target business pool of a new product for the first category and 1% for the second category.
As a rule of thumb: Complex systems contain more valuable insights simply because it’s hard for humans to see patterns in it but computers might be able to unveil them.
I’m writing this partly also because I believe, that starting the process of treating data properly contains a huge opportunity for all of us. It can contribute to improving processes and therefore stop wasting human capital. This is why I see using data properly as a corporate social responsibility.